Harnessing AI to Combat Microplastic Pollution Effectively
Written on
Chapter 1: Understanding Microplastic Pollution
Imagine minute particles of plastic, invisible to the naked eye, suspended in our water, food, and even within our lungs. These microplastics pose an escalating threat, as they are not only ubiquitous but also potentially detrimental to human health. Researchers are racing against time to grasp the implications of these pollutants, yet they encounter a formidable hurdle: insufficient data. This is where deep learning, a branch of artificial intelligence, typically excels, although it falters in the face of sparse or uneven data distribution.
Section 1.1: Introducing GANsemble
GANsemble functions like an elite team of superheroes, combining two potent AI methodologies: data augmentation and generative adversarial networks (GANs). Here's the breakdown of its operation. Initially, it identifies the most effective way to enhance existing data through a component known as the Data Chooser. This module tests various strategies to determine the optimal approach. Subsequently, it employs a conditional GAN (cGAN) to create new, synthetic data that is tailored to produce specific categories of microplastic data that are underrepresented in the original dataset. This synthetic data aids in bridging the gaps, resulting in AI models that are more robust and precise.
Subsection 1.1.1: The Data Chooser Module
The Data Chooser module marks the first stage in the GANsemble methodology. It takes a limited, imbalanced dataset along with a set of fundamental augmentation strategies, such as flipping or rotating images, and searches for the best combination. This process resembles a chef trying various recipes to achieve the ideal dish. The module assesses each combination using a pre-trained model, selecting the one that optimizes the model's performance. This chosen strategy is then utilized to train the cGAN, which generates the synthetic data.
Section 1.2: The cGAN Module and SYMP-Filter
After identifying the best augmentation method, the cGAN module takes charge. It leverages this strategy to train a generator that produces synthetic images of microplastics. These generated images are then evaluated by a discriminator, which learns to differentiate between real and synthetic data, refining the generator's output. To further enhance the quality of these synthetic images, GANsemble incorporates a SYMP-Filter. This algorithm eliminates noisy and less accurate synthetic images, ensuring that only high-quality data is used for training.
Chapter 2: Real-World Applications of GANsemble
The capability of GANsemble to generate superior synthetic data is revolutionary for research on microplastics. By addressing data gaps, it enables researchers to train more precise models, facilitating a deeper understanding and mitigation of the effects of microplastics on health and the environment. This innovation is not confined to microplastics; it can be utilized in any discipline where data is limited or unbalanced, establishing it as a versatile asset in the toolkit of AI researchers.
Below is a graph that visually represents the improvement in AI model performance with the use of GANsemble-generated synthetic data compared to traditional methods.
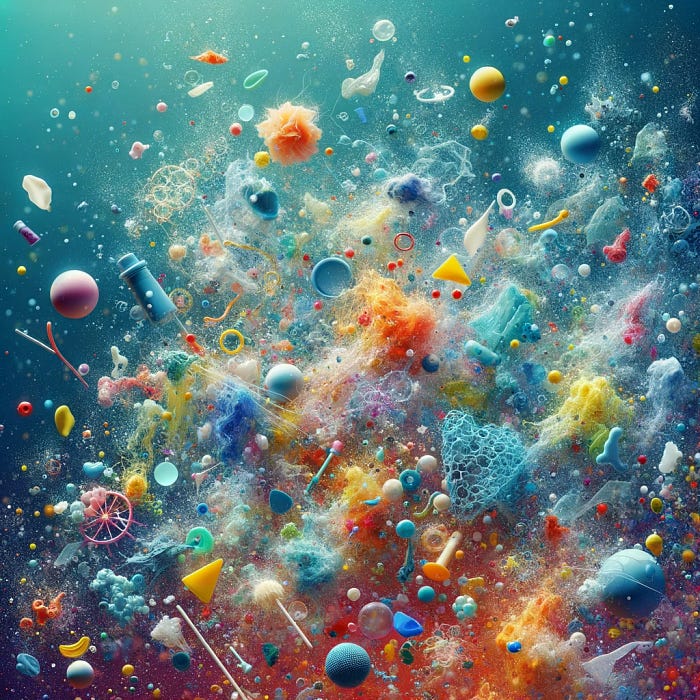
First Application to Microplastics
GANsemble is pioneering AI technology that creates synthetic data specifically for microplastics. This groundbreaking utilization sets a new benchmark for the application of AI in environmental research.
High-Quality Synthetic Data
The synthetic data produced by GANsemble is of such exceptional quality that it closely mimics real microplastics data, making it incredibly beneficial for training AI models.
Automated Augmentation
GANsemble's Data Chooser module automates the discovery of the best data augmentation strategy, saving researchers countless hours that would otherwise be spent on trial and error.
Versatility Across Domains
While originally designed for microplastics, GANsemble's methodology can be adapted to any field characterized by small or imbalanced datasets, spanning from medical research to financial forecasting.
Improved Model Performance
By generating more balanced and comprehensive datasets, GANsemble significantly enhances the performance of AI models, leading to more accurate predictions and insights.
A Bright Future with GANsemble
The advent of GANsemble signifies a substantial advancement in the battle against microplastics. By tackling the crucial issue of data scarcity, it opens new avenues for researchers, enabling more precise studies and effective solutions. This technology embodies the essence of innovation and progress, demonstrating that even the most formidable challenges can be surmounted through creativity and determination. For aspiring scientists and inquisitive minds, GANsemble represents a beacon of hope, showcasing the remarkable potential of AI to positively influence the world. The future appears promising, and with innovations like GANsemble, it is destined to shine even brighter.