Lyft's Comprehensive Machine Learning Platform: Flyte Explained
Written on
Chapter 1: Introduction to Flyte
As Lyft expanded its operations, managing intricate machine learning (ML) and data pipelines became increasingly challenging. The convergence of data and machine learning processes necessitated a unified tool to simplify this complexity. In response, Lyft developed and open-sourced Flyte, a cloud-native platform that integrates data and machine learning operations seamlessly.
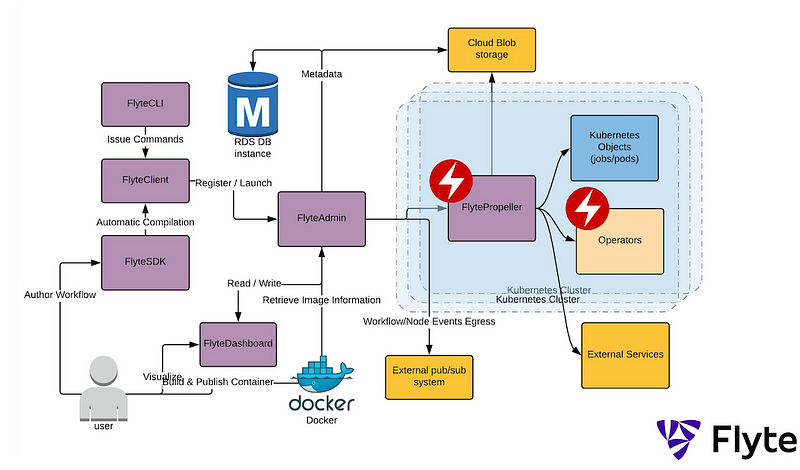
Chapter 2: The Architecture of Flyte
Why was Flyte Developed?
Lyft designed Flyte to effectively orchestrate ML and data workflows. A key objective was to facilitate collaboration and reusability across teams while optimizing ML operations. Consequently, Flyte serves as a scalable, serverless orchestration platform that connects various computing technologies.
How Does Flyte Function?
Built on Kubernetes, Flyte supports at least 7,000 unique workflows, executing over 100,000 tasks each month, which translates to managing 1 million tasks and 10 million containers. The immutability of all entities within Flyte allows for precise tracking of data lineage, replication of experiments, and rollback capabilities for deployments. Additionally, repeated tasks can utilize a cache system to enhance efficiency and reduce costs. Flyte currently accommodates tasks in Python, Hive, Presto, and Spark, along with supplementary sidecar functionalities.
Chapter 3: Data Cataloging in Flyte
Every task execution in Flyte is logged by default within the catalog service, creating an artifact lineage that traces causal relationships between data and processes. Memoization is also implemented, where each task execution is assigned a unique signature containing input values and code versions. This allows repeated executions with matching signatures to be cached, benefiting the team.
Flytekit Plugins and Backend Customization
Flytekit provides straightforward extensibility, handling boilerplate code while offering tools for development, testing, and deployment. These plugins operate within containers, allowing for rapid capability enhancements of Flyte. The backend is also designed for extensibility through a simple Golang interface available under FlytePlugins.
Notebooks and Papermill Integration
Flytekit enables the creation of various task types (Spark, Hive, Python, etc.) from Python notebooks, complete with comprehensive input/output functionalities. Papermill notebooks can be executed with any kernel, utilizing primitive inputs and outputs. The integration allows for seamless transitions from development to production while ensuring robust debugging options.
Chapter 4: Conclusion
Flyte encompasses all essential elements of a robust engineering system, including extensibility, observability, security, and auditing capabilities. Moreover, it integrates premier open-source solutions to address challenges in data and machine learning.
If you’re keen on enhancing your knowledge in machine learning, consider subscribing to our Acing AI newsletter for valuable insights.
Newsletter
Join the Acing AI/Data Science Newsletter for FREE! Stay updated on the latest trends in data science and machine learning.
www.acingdatascienceinterviews.com
If you are eager to master machine learning interviews, explore our course on acing data science interviews.
Acing Data Science Course
Enhance your skills with our comprehensive course on acing data science interviews.
www.acingdatascienceinterviews.com